The number of significant new AI models coming out of industry has surged in recent years relative to academia and government.
Stanford HAI
The seventh-annual report on the global state of artificial intelligence from Stanford University’s Institute for Human-Centered Artificial Intelligence offers some concerning thoughts for society: the technology’s spiraling costs and poor measurement of its risks.
According to the report, “The AI Index 2024 Annual Report,” published Monday by HAI, the cost of training large language models such as OpenAI’s GPT-4 — the so-called foundation models used to develop other programs — is soaring.
Also: Dana-Farber Cancer Institute finds main GPT-4 concerns include falsehoods, high costs
“The training costs of state-of-the-art AI models have reached unprecedented levels,” the report’s authors write. “For example, OpenAI’s GPT-4 used an estimated $78 million worth of compute to train, while Google’s Gemini Ultra cost $191 million for compute.”
(An “AI model” is the part of an AI program that contains numerous neural net parameters and activation functions that are the key elements for how an AI program functions.)
At the same time, the report states, there is too little in the way of standard measures of the risks of such large models because measures of “responsible AI” are fractured.
<!–>
There is “significant lack of standardization in responsible AI reporting,” the report states. “Leading developers, including OpenAI, Google, and Anthropic, primarily test their models against different responsible AI benchmarks. This practice complicates efforts to systematically compare the risks and limitations of top AI models.”
Both issues, cost and safety, are part of a burgeoning industrial market for AI, especially Gen AI, where commercial interests, and real-world deployments, are taking over from what has for many decades been mostly a research community of AI scholars.
Also: OpenAI’s stock investing GPTs fail this basic question about stock investing
“Investment in generative AI skyrocketed” in 2023, the report notes, as the industry produced 51 “notable” machine learning models – vastly more than the 15 that came out of academia last year. “More Fortune 500 earnings calls mentioned AI than ever before.”
The 502-page report goes into substantial detail on each point. On the first point – training cost – the report’s authors teamed up with research institute Epoch AI to estimate the training cost of foundation models. “AI Index estimates validate suspicions that in recent years model training costs have significantly increased,” the report states.
For example, in 2017, the original Transformer model, which introduced the architecture that underpins virtually every modern LLM, cost around $900 to train. RoBERTa Large, released in 2019, which achieved state-of-the-art results on many canonical comprehension benchmarks like SQuAD and GLUE, cost around $160,000 to train. Fast-forward to 2023, and training costs for OpenAI’s GPT-4 and Google’s Gemini Ultra are estimated to be around $78 million and $191 million, respectively.
The report notes that training costs are rising with the increasing size of computation required for the increasingly large AI models. The original Google Transfomer, the deep learning model that sparked the race for GPTs and other large language models, required about 10,000 petaFLOPs, or 10,000 trillion floating point operations. Gemini Ultra approaches a hundred billion petaFLOPs.
At the same time, assessing the AI programs for their safety – including transparency, explainability, and data privacy – is difficult. There has been a proliferation of benchmark tests to assess “responsible AI,” and developers are using many of them so that there isn’t consistency. “Testing models on different benchmarks complicates comparisons, as individual benchmarks have unique and idiosyncratic natures,” the report states. “New analysis from the AI Index, however, suggests that standardized benchmark reporting for responsible AI capability evaluations is lacking.”
Also: As AI agents spread, so do the risks, scholars say
The AI Index examined a selection of leading AI model developers, specifically OpenAI, Meta, Anthropic, Google, and Mistral AI. The Index identified one flagship model from each developer (GPT-4, Llama 2, Claude 2, Gemini, and Mistral 7B) and assessed the benchmarks on which they evaluated their model. A few standard benchmarks for general capabilities evaluation were commonly used by these developers, such as MMLU, HellaSwag, ARC Challenge, Codex HumanEval, and GSM8K. However, consistency was lacking in the reporting of responsible AI benchmarks. Unlike general capability evaluations, there is no universally accepted set of responsible AI benchmarks used by leading model developers.
A table of benchmarks reported by the models shows a great variety but no consensus on which benchmarks for responsible AI should be considered standard.
“To improve responsible AI reporting,” the authors conclude, “it is important that a consensus is reached on which benchmarks model developers should consistently test.”
Also: Cybercriminals are using Meta’s Llama 2 AI, according to CrowdStrike
On a positive note, the study’s authors emphasize that data shows AI is having a positive impact on productivity. “AI enables workers to complete tasks more quickly and to improve the quality of their output,” the research shows.
Specifically, the report notes that professional programmers saw their rates of project completion increase with the help of AI, according to a review last year by Microsoft. The review found that “comparing the performance of workers using Microsoft Copilot or GitHub’s Copilot – LLM-based productivity-enhancing tools – with those who did not, found that Copilot users completed tasks in 26% to 73% less time than their counterparts without AI access.”
Increased ability was found in other labor groups, according to other studies. A Harvard Business School report found “consultants with access to GPT-4 increased their productivity on a selection of consulting tasks by 12.2%, speed by 25.1%, and quality by 40%, compared to a control group without AI access.”
Also: Can enterprise identities fix Gen AI’s flaws? This IAM startup thinks so
The Harvard study also found that less-skilled consultants saw a bigger boost from AI, in terms of improved performance on tasks, than did more skillful counterparts, suggesting that AI helps to close a skills gap.
“Likewise, National Bureau of Economic Research research reported that call-center agents using AI handled 14.2% more calls per hour than those not using AI.”
Despite the risk of things such as “hallucinations,” legal professionals using OpenAI’s GPT-4 saw benefits “in terms of both work quality and time efficiency across a range of tasks” including contract drafting.
There is a downside to productivity, however. Another Harvard paper found that the use of AI by professional talent recruiters impaired their performance. Worse, those using more powerful AI tools seemed to see even greater degradation in their job performance. The study theorizes that recruiters using “good AI” became complacent, overly trusting the AI’s results, unlike those using “bad AI,” who were more vigilant in scrutinizing AI output.
Study author Fabrizio Dell’Acqua of Harvard Business School dubs the phenomenon of complacency amidst AI use as “falling asleep at the wheel.”
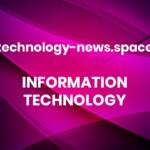
XZ Utils might not have been the only sabotage target, open-source foundations warn
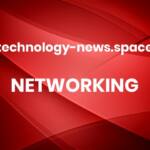